Setup Latest JAX/CUDA on Ubuntu 22.04
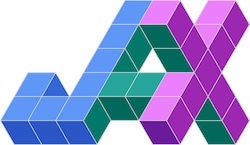
Table of Contents
Introduction⌗
JAX is this rad library from Google research that acts as a Domain Specific Language (DSL) for machine learning, similar to how Halide-lang is a DSL for image processing. It’s pretty hard to get it working properly on Ubuntu since there are a lot of easy pitfalls. However, this guide will get you up and running on the latest bleeding edge of everything on Ubuntu 22.04.
First remove previous Nvidia drivers⌗
There are two normal ways to install Nvidia drivers on Ubuntu that are familiar (1) Download the run-file from Ubuntu and manually install and (2) Use the nvidia-driver-515 package.
To uninstall the runfile version:
sudo bash NVIDIA-Linux-x86_64-XXX.XX.run --uninstall
To uninstall the Ubuntu package version:
sudo apt remove nvidia-driver-*
sudo apt autoremove
The autoremove is important to get rid of some files we need to overwrite next with symlinks.
Install cuda package⌗
Instructions are here: https://developer.nvidia.com/cuda-downloads?target_os=Linux&target_arch=x86_64&Distribution=Ubuntu&target_version=22.04&target_type=deb_network
wget https://developer.download.nvidia.com/compute/cuda/repos/ubuntu2204/x86_64/cuda-keyring_1.0-1_all.deb
sudo dpkg -i cuda-keyring_1.0-1_all.deb
sudo apt-get update
sudo apt-get -y install cuda cuda-11-8
sudo update-alternatives --set cuda /usr/local/cuda-11.8
sudo reboot now
Check which version of CUDA is installed:
(base) catid@nuc:~$ nvidia-smi
Mon Oct 17 00:17:59 2022
+-----------------------------------------------------------------------------+
| NVIDIA-SMI 520.61.05 Driver Version: 520.61.05 CUDA Version: 11.8 |
|-------------------------------+----------------------+----------------------+
| GPU Name Persistence-M| Bus-Id Disp.A | Volatile Uncorr. ECC |
| Fan Temp Perf Pwr:Usage/Cap| Memory-Usage | GPU-Util Compute M. |
| | | MIG M. |
|===============================+======================+======================|
| 0 NVIDIA GeForce ... On | 00000000:01:00.0 Off | N/A |
| 0% 54C P8 26W / 350W | 1MiB / 24576MiB | 0% Default |
| | | N/A |
+-------------------------------+----------------------+----------------------+
+-----------------------------------------------------------------------------+
| Processes: |
| GPU GI CI PID Type Process name GPU Memory |
| ID ID Usage |
|=============================================================================|
| No running processes found |
+-----------------------------------------------------------------------------+
You can see version 11.8 of CUDA is installed. Since we ran the up-date-alternatives command, we can now use the nvcc
compiler.
(base) catid@nuc:~$ nvcc --version
nvcc: NVIDIA (R) Cuda compiler driver
Copyright (c) 2005-2022 NVIDIA Corporation
Built on Wed_Sep_21_10:33:58_PDT_2022
Cuda compilation tools, release 11.8, V11.8.89
Build cuda_11.8.r11.8/compiler.31833905_0
Test CUDA installation⌗
git clone https://github.com/NVIDIA/cuda-samples.git
cd ./cuda-samples/Samples/1_Utilities/deviceQuery
vi Makefile
Set the CUDA_PATH=
line to install path of CUDA. Example:
CUDA_PATH ?= /usr/local/cuda-11.8
This path is important to note for when we install JAX later.
Build and run the tool:
make
./deviceQuery
(base) catid@nuc:~/cuda-samples/Samples/1_Utilities/deviceQuery$ ./deviceQuery
./deviceQuery Starting...
CUDA Device Query (Runtime API) version (CUDART static linking)
Detected 1 CUDA Capable device(s)
Device 0: "NVIDIA GeForce RTX 3090"
CUDA Driver Version / Runtime Version 11.8 / 11.8
CUDA Capability Major/Minor version number: 8.6
Total amount of global memory: 24268 MBytes (25447170048 bytes)
(082) Multiprocessors, (128) CUDA Cores/MP: 10496 CUDA Cores
GPU Max Clock rate: 1725 MHz (1.73 GHz)
Memory Clock rate: 9751 Mhz
Memory Bus Width: 384-bit
L2 Cache Size: 6291456 bytes
Maximum Texture Dimension Size (x,y,z) 1D=(131072), 2D=(131072, 65536), 3D=(16384, 16384, 16384)
Maximum Layered 1D Texture Size, (num) layers 1D=(32768), 2048 layers
Maximum Layered 2D Texture Size, (num) layers 2D=(32768, 32768), 2048 layers
Total amount of constant memory: 65536 bytes
Total amount of shared memory per block: 49152 bytes
Total shared memory per multiprocessor: 102400 bytes
Total number of registers available per block: 65536
Warp size: 32
Maximum number of threads per multiprocessor: 1536
Maximum number of threads per block: 1024
Max dimension size of a thread block (x,y,z): (1024, 1024, 64)
Max dimension size of a grid size (x,y,z): (2147483647, 65535, 65535)
Maximum memory pitch: 2147483647 bytes
Texture alignment: 512 bytes
Concurrent copy and kernel execution: Yes with 2 copy engine(s)
Run time limit on kernels: No
Integrated GPU sharing Host Memory: No
Support host page-locked memory mapping: Yes
Alignment requirement for Surfaces: Yes
Device has ECC support: Disabled
Device supports Unified Addressing (UVA): Yes
Device supports Managed Memory: Yes
Device supports Compute Preemption: Yes
Supports Cooperative Kernel Launch: Yes
Supports MultiDevice Co-op Kernel Launch: Yes
Device PCI Domain ID / Bus ID / location ID: 0 / 1 / 0
Compute Mode:
< Default (multiple host threads can use ::cudaSetDevice() with device simultaneously) >
deviceQuery, CUDA Driver = CUDART, CUDA Driver Version = 11.8, CUDA Runtime Version = 11.8, NumDevs = 1
Result = PASS
Install cuDNN⌗
Download the .deb file from https://developer.nvidia.com/rdp/cudnn-download
This requires an Nvidia developer account.
I downloaded and installed this file:
sudo dpkg -i cudnn-local-repo-ubuntu2204-8.6.0.163_1.0-1_amd64.deb
sudo cp /var/cudnn-local-repo-ubuntu2204-8.6.0.163/cudnn-local-FAED14DD-keyring.gpg /usr/share/keyrings/
sudo apt install libcudnn8-dev
This seems to put the cudnn library headers/files under /usr
.
Easy install jax⌗
This seems to work fine:
pip install --upgrade "jax[cuda]" -f https://storage.googleapis.com/jax-releases/jax_cuda_releases.html
Manual build and install jaxlib⌗
These steps are from https://jax.readthedocs.io/en/latest/developer.html#building-from-source
Build jaxlib:
git clone https://github.com/google/jax
cd jax
sudo apt install g++ python3 python3-dev
pip install numpy wheel
python build/build.py --enable_cuda --cuda_path /usr/local/cuda-11.8 --cudnn_path /usr
The build takes about 30 minutes on a modern processor. Finally, time to install!
pip install --upgrade pip
pip install dist/*.whl
pip install -e . # installs jax
Test JAX⌗
Write a simple test Python script from the Jax repo https://github.com/google/jax
from jax import grad
import jax.numpy as jnp
def tanh(x): # Define a function
y = jnp.exp(-2.0 * x)
return (1.0 - y) / (1.0 + y)
grad_tanh = grad(tanh) # Obtain its gradient function
print(grad_tanh(1.0)) # Evaluate it at x = 1.0
# prints 0.4199743
Run the test:
(base) catid@nuc:~$ python temp.py
0.4199743
I used tmux
and watch nvidia-smi
in a second pane to verify that the GPU was actually getting used for this script.